Understanding Machine Learning: Empowering Your Business
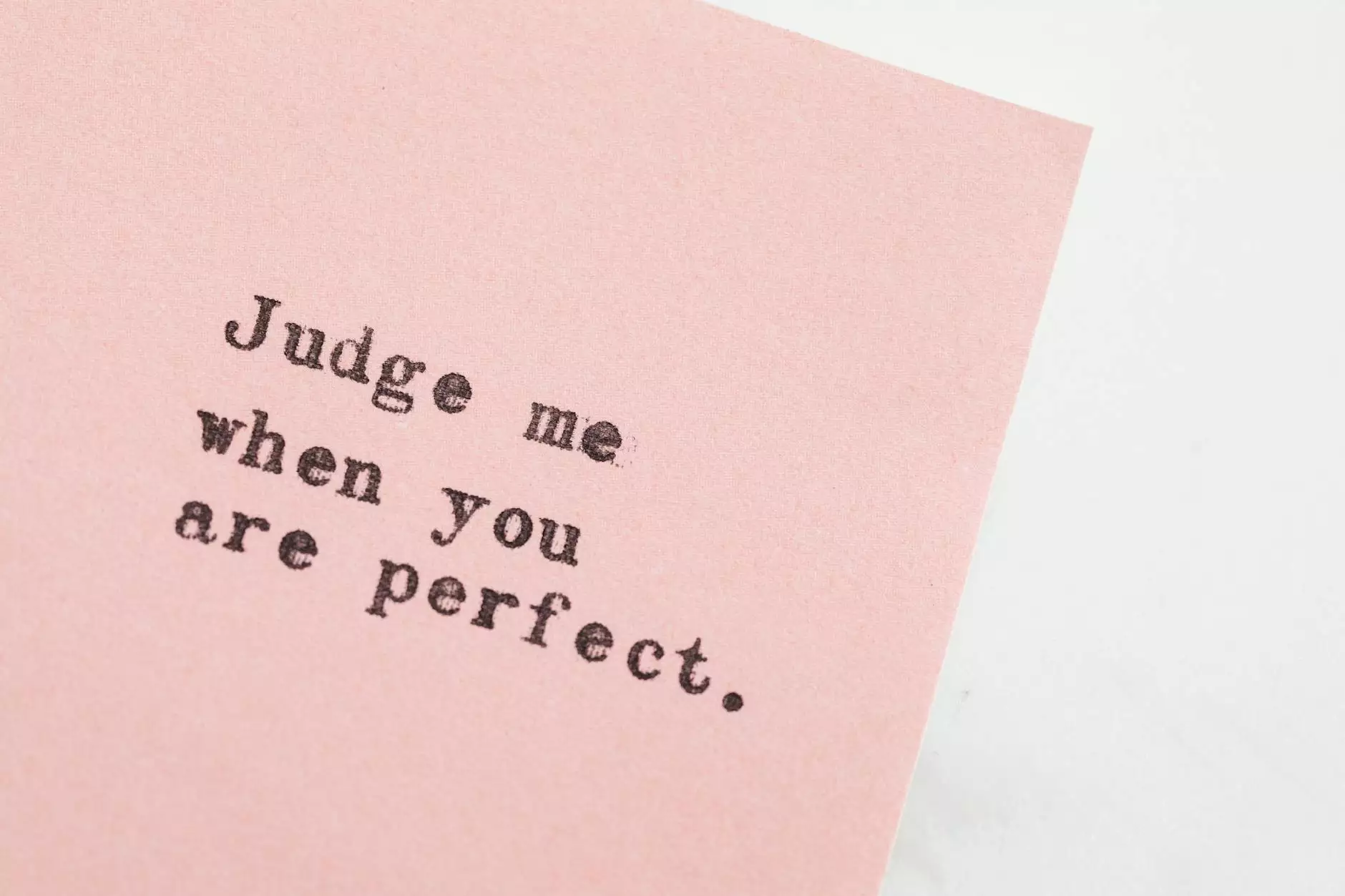
Machine Learning is revolutionizing the way businesses operate, analyze data, and make decisions. In an era defined by rapid technological advancement, the integration of machine learning into business strategies is not just beneficial, but essential. This comprehensive article delves deeply into the various aspects of machine learning, its applications in business, and the profound impact it can have on organizations worldwide.
The Fundamentals of Machine Learning
At its core, machine learning is a subset of artificial intelligence that enables systems to learn from data, improve their accuracy over time, and make predictions without being explicitly programmed to do so. Machine learning algorithms analyze existing data to identify patterns and trends, which can then be used to inform decision-making processes.
Key Concepts in Machine Learning
Understanding machine learning involves familiarizing oneself with several key concepts:
- Supervised Learning: This involves training a model on a labeled dataset, meaning that the input data is paired with the correct output. Examples include classification and regression tasks.
- Unsupervised Learning: In contrast, unsupervised learning uses data that has no labels, allowing the algorithm to infer the natural structure present within the dataset through clustering or association.
- Reinforcement Learning: This type of learning involves training agents to make a series of decisions by rewarding desired actions and punishing undesired ones, commonly used in robotics and gaming.
- Deep Learning: A specialized form of machine learning that uses neural networks with many layers (hence "deep"). It excels in handling vast amounts of unstructured data like images and text.
Applications of Machine Learning in Business
The applications of machine learning in business are vast and varied. Companies across industries are leveraging this technology to enhance their operations, drive efficiency, and gain a competitive edge. Below, we explore the most impactful applications of machine learning in the business landscape.
1. Predictive Analytics
Predictive analytics harnesses machine learning algorithms to analyze trends within data, enabling businesses to make informed decisions based on future predictions. For instance, retailers can anticipate customer purchasing behaviors, optimize stock levels, and personalize marketing strategies.
2. Customer Service Automation
Machine learning is transforming customer service through the deployment of chatbots and virtual assistants. These tools can provide instant responses to customer inquiries, process transactions, and gather insights on customer preferences. This not only improves customer satisfaction but also reduces operational costs.
3. Fraud Detection
Financial institutions are employing machine learning algorithms to detect fraudulent activities. By analyzing transaction patterns in real-time, these systems can flag unusual behaviors and significantly reduce the risk of fraud.
4. Product Recommendation Systems
Machine learning powers recommendation engines that suggest products to users based on their past behaviors and preferences. Companies like Amazon and Netflix utilize these systems to enhance user engagement and boost conversions.
5. Human Resource Management
In the realm of human resources, machine learning can streamline recruitment processes by analyzing resumes and identifying candidates who best fit the job descriptions, thereby reducing time-to-hire and improving the quality of hires.
The Business Value of Machine Learning
The integration of machine learning into business processes can yield considerable value. Here are several ways in which machine learning about business can enhance corporate performance:
- Increased Efficiency: Automation of repetitive tasks frees up employees to focus on higher-value work.
- Enhanced Decision Making: Data-driven insights lead to better strategic decisions and optimized operations.
- Cost Reduction: Improved processes and the ability to predict maintenance needs can lead to significant savings.
- Customer Insights: Analyzing data helps businesses understand their customers better, leading to improved products and services tailored to customer needs.
- Innovation: Machine learning enables the development of new products and services that can meet emerging customer demands.
Implementing Machine Learning in Your Business
For businesses looking to adopt machine learning, a strategic approach is essential. Here are the steps to effectively implement machine learning:
1. Define Business Problems
The first step is to identify the specific business problems that machine learning can solve. Clarifying objectives ensures that efforts are focused and relevant.
2. Data Collection
Gather relevant data that can be used for training machine learning models. Data quality is critical; poor data can lead to inaccurate models.
3. Model Selection
Choose the appropriate machine learning algorithms based on the problem at hand. Different models serve various purposes, so it's crucial to select the right one.
4. Training the Model
Train the selected model using the collected data. This involves processing the data and adjusting the model parameters to improve accuracy.
5. Testing and Validation
After training, the model must be tested with unseen data to validate its performance. It's important to ensure that the model generalizes well to new data.
6. Deployment
Once validated, deploy the model into the business operational processes where it can contribute to decision-making and enhance efficiency.
7. Monitoring and Maintenance
Continuous monitoring of the model's performance is essential. Regular updates and retraining may be necessary to adapt to new data and changing patterns.
Challenges of Machine Learning in Business
While machine learning offers immense potential, it is not without challenges. Understanding these obstacles is vital for successful implementation:
- Data Privacy Concerns: The collection and use of personal data can lead to privacy concerns. Businesses must ensure compliance with regulations like GDPR.
- Integration with Existing Systems: Integrating machine learning systems with legacy systems can be complex and resource-intensive.
- Skill Gaps: There is a significant demand for data scientists and machine learning engineers, leading to a skill gap that many organizations struggle to fill.
- Bias in Data: Ensuring that models do not inherit biases from training data is crucial for ethical machine learning practices.
Future Trends in Machine Learning
The future of machine learning in business is bright, with numerous trends set to shape its evolution:
- Explainable AI: Developing algorithms that provide clear insights into their decision-making processes is critical for gaining user trust.
- Edge Computing: Processing data on devices rather than central servers will enable faster decision-making and lower latency.
- Automated Machine Learning (AutoML): Tools that automate the selection, training, and evaluation of machine learning models are making it accessible to non-experts.
- Augmented Analytics: Combining machine learning with natural language processing and augmented analytics tools will enhance data visualization and interpretation.
Conclusion
As we continue to explore the realm of machine learning about business, it is clear that this technology is more than just a trend; it is a vital component of modern business strategy. By understanding its applications, benefits, and challenges, organizations can leverage machine learning to drive innovation, enhance efficiency, and ultimately increase profitability.
Embracing machine learning unfolds a world of opportunities, enabling businesses to stay ahead of the curve in today's competitive landscape. Whether you own a small business or manage a multinational corporation, investing in machine learning technologies is a step toward future readiness and sustained success.