Revolutionizing Machine Learning with Effective Video Annotation Tools
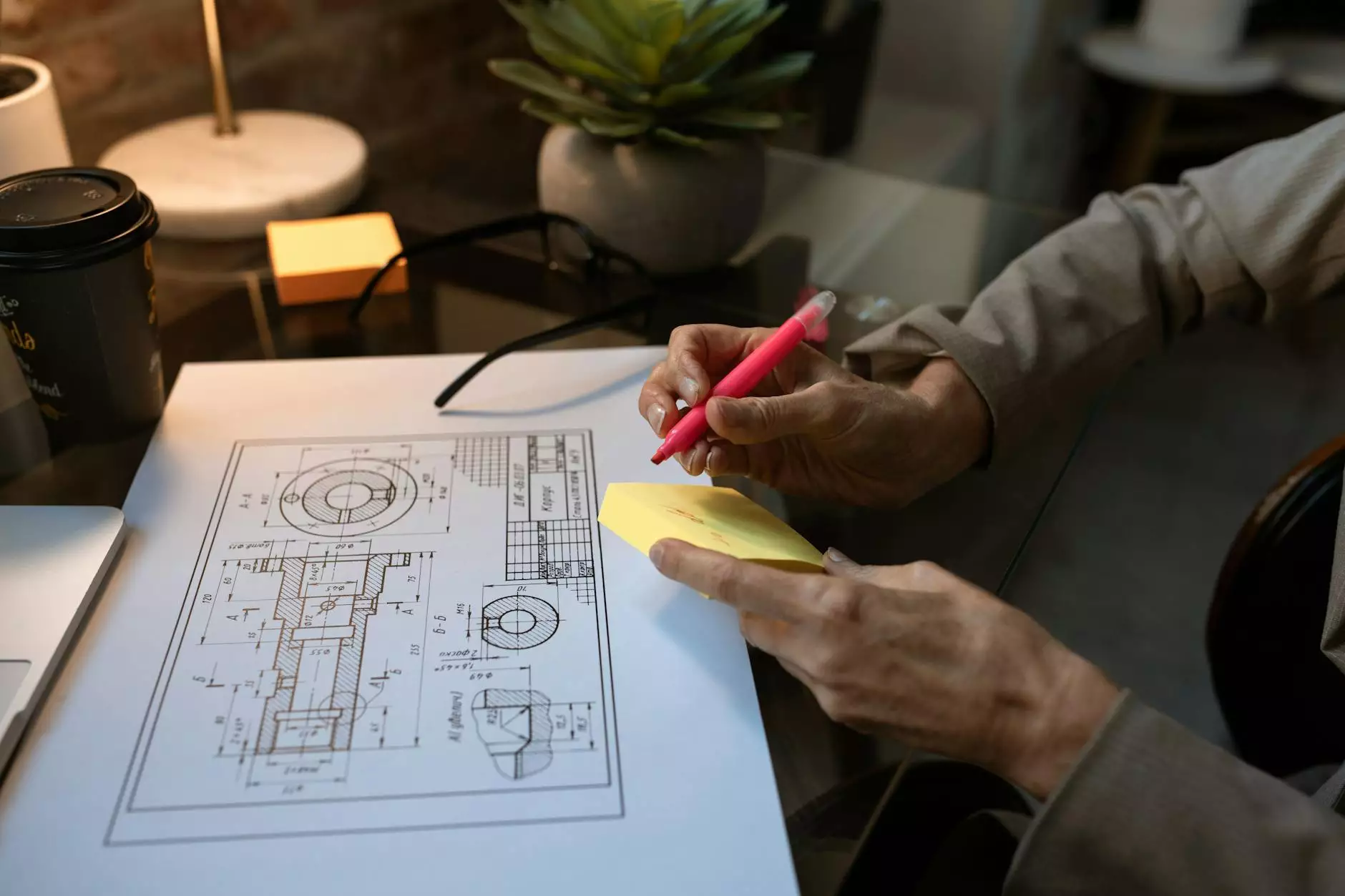
In the rapidly evolving landscape of machine learning, the need for high-quality training data cannot be overstated. One of the critical aspects of creating effective machine learning models involves accurate data annotation. This is particularly true for video data, which presents unique challenges and opportunities. Enter the video annotation tool for machine learning, a revolutionary solution that enhances the data annotation process, ensuring that machine learning algorithms can learn from well-structured, comprehensive datasets.
Understanding Video Annotation and Its Importance
Video annotation is the process of labeling and marking specific elements within video clips, enabling machine learning models to learn from this data. With the exponential growth of video content being produced globally, the demand for quality annotation tools has surged. These tools facilitate the identification of objects, activities, and interactions, which are essential for tasks such as:
- Facial Recognition: Identifying faces in security applications.
- Activity Detection: Tracking movements for health monitoring.
- Object Tracking: Following specific items in real-time for surveillance.
- Autonomous Vehicles: Teaching vehicles to navigate and recognize road signs.
Key Features of Video Annotation Tools
To maximize the efficiency and accuracy of video annotation, a good video annotation tool for machine learning should incorporate several essential features:
1. Intuitive User Interface
A user-friendly interface ensures that annotators can work quickly and effectively, minimizing the learning curve. Many advanced tools offer drag-and-drop functionalities and customizable layouts that cater to different projects and user preferences.
2. Advanced Annotation Capabilities
Robust tools support various types of annotations, including:
- Bounding Boxes: Marking specific areas within a video frame.
- Polylines: Drawing complex shapes for detailed annotations.
- Semantic Segmentation: Classifying each pixel in an image for thorough understanding.
- 3D Point Clouds: Capturing depth information for volumetric analysis.
3. Collaboration Features
Many video annotation projects require teamwork. Tools that offer real-time collaboration and easy sharing options can significantly boost productivity and ensure that all team members are on the same page.
4. Quality Control Mechanisms
Ensuring the accuracy of annotations is vital. Integrated quality control features allow teams to review, edit, and validate annotations. These mechanisms can help catch errors and improve the overall quality of the training data.
5. Scalability
As projects grow, the amount of data that needs to be annotated can increase significantly. A scalable video annotation tool can handle vast datasets without compromising speed or quality, making it ideal for large-scale machine learning projects.
The Benefits of Using Video Annotation Tools
Investing in a video annotation tool for machine learning can provide significant advantages for businesses and researchers alike. Here are some of the primary benefits:
Enhanced Data Quality
High-quality annotated data is crucial for training reliable machine learning models. Effective video annotation tools help ensure that the data fed into these models is accurate and well-structured, leading to better performance and more reliable outcomes.
Time Efficiency
Manual annotation can be time-consuming. Advanced tools automate many aspects of the annotation process, significantly reducing the time required to prepare datasets. This means that teams can focus on refinement and analysis rather than just data tagging.
Cost-Effectiveness
While there may be initial costs associated with high-end annotation tools, the long-term savings in time and improved model efficiency can lead to substantial overall cost reductions in machine learning project budgets.
Greater Insights and Outcomes
Detailed and accurate annotations allow machine learning models to yield better insights. Businesses can leverage this improved performance to enhance decision-making processes and improve customer experiences across various applications.
Applications of Video Annotation Tools in Various Industries
The versatility of video annotation tools makes them valuable across numerous industries. Here are a few significant applications:
1. Healthcare
In healthcare, video annotation can aid in patient monitoring and treatment analysis. For instance, annotated videos can help in analyzing patient movements for rehabilitation purposes or in identifying irregularities in patient behavior.
2. Automotive
In the automotive sector, video annotation is crucial for developing autonomous driving technology. These tools help train models to recognize road signs, pedestrians, and other vehicles, leading to safer navigation systems.
3. Security and Surveillance
Enhanced video surveillance systems utilize annotation tools to track and analyze activities within secure environments. Properly annotated video data can improve anomaly detection and reduce false alarms, ultimately enhancing safety protocols.
4. Sports Analytics
Sports organizations employ video annotation tools to analyze player performance, tactics, and strategies. By dissecting game footage with fine detail, coaches can develop better training programs and improve overall team performance.
Choosing the Right Video Annotation Tool
Given the plethora of options available, selecting the right video annotation tool for machine learning can be a challenging decision. Here are some factors to consider when making your choice:
1. Purpose and Use Case
Identify the specific objectives of your project. Different tools may be optimized for various use cases, such as tracking, classification, or segmentation. Ensure that the tool you choose aligns with your project goals.
2. Budget
Consider both upfront costs and potential long-term savings. Some tools may have subscription models, while others require a one-time investment. Assessing the total cost of ownership helps in making a more informed choice.
3. Support and Resources
Evaluate the availability of customer support and learning resources. A good annotation tool should come with comprehensive documentation, tutorials, and customer support to help you maximize its potential.
4. Integration Capabilities
Ensure that the tool can seamlessly integrate with your existing workflows and systems. Compatibility with popular machine learning frameworks is essential for a smooth operation.
Conclusion
Investing in a video annotation tool for machine learning is a transformative step that can significantly enhance the quality of training data, thereby improving the performance of machine learning models. By adopting these tools, businesses and researchers can streamline their annotation processes, save time, and ultimately achieve better results. Whether in healthcare, automotive, security, or sports analytics, the benefits of effective video annotation tools are evident. As machine learning continues to advance, the role of precision in data annotation cannot be underestimated, making these tools indispensable in the journey toward innovation.
For more information on how to leverage the best video annotation tools for your machine learning projects, visit keylabs.ai.