Unlocking the Power of Data with a Superior Data Labelling Tool
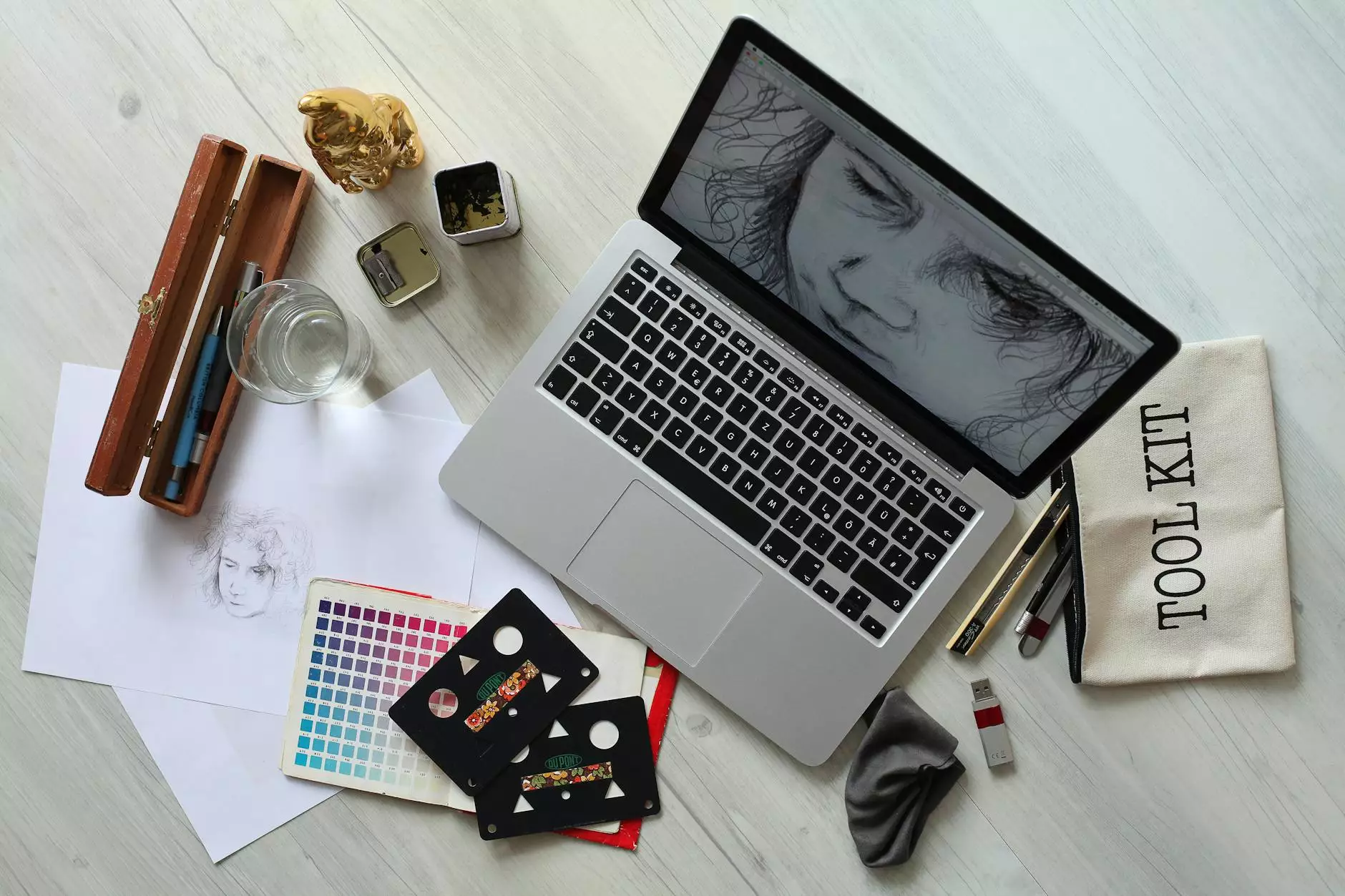
In the age of artificial intelligence and machine learning, the importance of accurate, high-quality data cannot be overstated. Central to this is the process of data labelling, which serves as the foundation for training intelligent systems. A reliable data labelling tool plays a pivotal role in transforming raw data into structured, usable formats, enhancing the performance and effectiveness of AI models. This comprehensive guide dives deep into the world of data labelling tools, why they are essential, and how to choose the right platform for your needs.
What is Data Labelling?
Data labelling refers to the process of identifying and tagging raw data with relevant labels or annotations. This process can be applied to various types of data including images, text, videos, and audio. The labelled data is then used to train machine learning models, helping them to make accurate predictions or classifications.
The Importance of Data Labelling in AI Development
As AI technologies continue to evolve, the effectiveness of these systems heavily relies on the quality of the training data. Here’s why data labelling is crucial:
- Quality Predictions: High-quality labelled data enhances the accuracy of machine learning models, reducing the chances of errors.
- Model Performance: Well-labelled datasets contribute to better model generalization, allowing AI systems to perform effectively on unseen data.
- Compliance and Ethics: Correct labelling ensures that bias is minimized, meeting ethical standards and legal regulations.
Types of Data Labelling Tools
Choosing the right data labelling tool can significantly impact your project. Various tools cater to different types of data and use cases. The common types include:
- Image Annotation Tools: These tools focus on tagging objects within images, used extensively in computer vision.
- Text Annotation Tools: Used for linguistic datasets, these tools label entities, sentiments, or parts of speech.
- Video Annotation Tools: These allow users to label specific frames in video data for action recognition or object tracking.
- Audio Annotation Tools: Used for transcription and tagging of audio files, essential in speech recognition applications.
Key Features of an Effective Data Labelling Tool
When selecting a data labelling tool, consider the following key features:
- Ease of Use: The tool should have a user-friendly interface, enabling quick onboarding for users.
- Collaboration Capabilities: Look for platforms that support team collaboration, allowing multiple annotators to work efficiently.
- Customizability: The ability to create custom labels or workflows can greatly enhance the tool's effectiveness.
- Integration: Ensure the tool can be integrated with your existing data processing and machine learning pipelines.
- Scalability: A good tool should handle projects of varying sizes, from small datasets to extensive collections.
Top Data Labelling Tools Available Today
Here are some of the leading data labelling tools available on the market:
1. Keylabs.ai
Keylabs.ai offers a powerful data annotation platform that provides a suite of tools for effective data labelling. It stands out for its intuitive interface, allowing users to easily annotate various data types from images to text. Keylabs.ai excels with its collaborative features, enabling teams to work seamlessly on projects while ensuring high accuracy and consistency in labelling.
2. Amazon SageMaker Ground Truth
Amazon SageMaker Ground Truth is a fully managed data labelling service that makes it easy to build highly accurate training datasets for machine learning. It offers tools for automating the labelling process, significantly reducing the time and cost involved.
3. Labelbox
Labelbox is a highly flexible and scalable data labelling tool that integrates well with various machine learning workflows. It provides an inclusive environment for teams to collaborate on data annotation, enhancing productivity significantly.
4. Supervisely
Supervisely is an all-in-one AI development platform that includes powerful data labelling capabilities. It is particularly advantageous for computer vision projects, offering extensive functionality for image and video annotation.
How to Choose the Right Data Labelling Tool
Choosing the right data labelling tool can be a daunting task given the myriad of options available. To make the selection process easier, follow these guidelines:
1. Define Your Needs
Start by identifying the specific requirements of your project. Consider the type of data you are working with and the kind of annotations you need.
2. Test the Tool
Most data labelling tools offer free trials or demos. Use these to assess usability and features that align with your project goals.
3. Evaluate Support and Documentation
Check for comprehensive user support and documentation. A tool with good support can save you time and frustration during implementation and usage.
4. Review Pricing Models
Understand the pricing structure—whether it's pay-per-use, subscription-based, or one-time fees. Choose a model that fits your budget and project scope.
Challenges in Data Labelling
While data labelling is essential, it comes with its own set of challenges. Here are some common issues faced in the industry:
- Time-Consuming Process: Manual data labelling can take a significant amount of time, impacting project timelines.
- Quality Assurance: Ensuring the accuracy and consistency of labels can be difficult, especially in large datasets.
- High Costs: Depending on the complexity and amount of data, costs can escalate quickly, especially if outsourcing is involved.
Future Trends in Data Labelling
The field of data labelling is constantly evolving. Some key trends to watch for include:
- Automation: The future will see increased automation in the labelling process, with AI-driven tools assisting in reducing manual effort.
- Crowdsourcing: Utilizing crowdsourced labour for data annotation can help manage costs and time effectively.
- Integration with Machine Learning: Tools that seamlessly integrate with machine learning frameworks will become more popular, enhancing the workflow.
Conclusion
As businesses increasingly rely on AI-driven solutions, the demand for efficient and effective data labelling tools will continue to grow. Investing in a top-quality data labelling tool, such as those offered by Keylabs.ai, not only enhances the quality of your datasets but also significantly boosts the overall performance of your AI applications. Whether you're a large enterprise or a budding startup, choosing the right annotation platform can set the foundation for successful AI system development. Stay ahead of the curve by understanding the importance of data labelling and leveraging the right tools for your specific needs.